State of Quantum Computing - Summer 2023
There is much discussion in advanced breakthroughs in computing lately and quantum computing (QC) has been a topic of interest. This article will go through the latest QC advances and consider how well it performs on real world problems.
There is much discussion in advanced breakthroughs in computing lately and quantum computing (QC) has been a topic of interest. This article will go through the latest QC advances and consider how well it performs on real world problems.
What is Quantum Computing?
QC is a form of computing which utilizes the principles of quantum mechanics to process and store data, allowing for faster and more efficient problem solving than traditional computing models. It harnesses the power of quantum physics to exploit the power of particles, exploiting their complex interactions to carry out computations that would be impossible for classical computers.
In contrast to traditional computers, which are constructed from bits, also known as ones and zeros, the fundamental unit of information in quantum computing is referred to as a quantum bit (or "qubit" for short). A "bit" is analogous to a gate in an electronic circuit, in that it can either be on or off. On the other hand, a qubit makes use of the special properties of quantum mechanics to provide a unit that can either be one or zero, or anything in between. The operations of superposition, entanglement, and interference are not present in the classical realm; however, these concepts are included in the rules of quantum mechanics. By developing algorithms that are able to take advantage of these effects, it is possible to find a solution to problems that would, in other circumstances, require a significant amount of traditional computing resources.
Why Does it Matter?
As Richard Feynman stated, "Nature is not classical so if you want to simulate nature you must make it quantum mechanical." Quantum computing seeks to mimic nature in order to calculate and solve problems in ways that classical computing cannot. This offers the potential to optimize very challenging problems that we cannot even approach today.
The Race for Quantum Computing
Progress in quantum computing has been slow, but the risk of being left out is great. This risk has generated an arms race amongst Nations and companies. There are 46 countries engaged in some form of quantum computing research, most in academia and industry. Many big companies are in the race too, firms like IBM, Google, Microsoft, and Honeywell are all building QC hoping to be the first to sell the mainstream quantum computing machine.
It is a very nascent field where each milestone offers big promises, but so far it has not delivered. For example, a major milestone was quantum computing demonstrating supremacy over traditional computers, but once it was achieved (an amazing event) it was realized that it accomplished little real world value. Much like Palantir's explanation of how to harness the power of Generative LLM AI models, ([[Palantir's View on Generative AI]]) using frameworks and systems of systems, it appears the same is true of quantum computing.
How to Quantum Compute
To solve problems with QC a critical component is the Quantum Algorithm. A quantum algorithm is a collection of quantum circuits that are designed to be executed on a quantum computer in order to solve a particular problem. The fundamental components that power quantum applications are called quantum algorithms. A single algorithm can serve as the building block for multiple applications across a variety of fields. But building these components is as very specialized and expensive.
Given the high physical infrastructural requirements to create environments in which QC operate, most users will get access to the technology via the cloud, and likely paired with other traditional cloud computing services. This also means that most commercial systems will be (or are) offered “as-a-service”, in collaboration with leading cloud providers. This means that organizations will likely be buying computing time, not QC. In the near future IBM is to offer a fledgling service called HERON that offers a modular QC "in the cloud" service.
This cloud design is hoped to mainstream implementation of quantum computing by providing quantum accelerators. Quantum accelerators would be similar to GPUs and how they are used in computing currently. This would allow systems to operate per the normal architectures but augment their capabilities with quantum accelerators. This would provide quantum level computing for most organizations.
IBM Heron
It is anticipated that IBM will launch its Heron processor this year (2023). This particular model will only have 133 qubits. Heron's qubits will be of the highest quality, and each chip will be able to connect directly to other Heron processors. This will mark the beginning of a transition away from single quantum computing chips and toward "modular" QC that are constructed from multiple processors that are connected together. Heron is a sign of larger shifts in the industry of quantum computing, and experts suggest that general-purpose QC may be seen sooner than many would have anticipated in light of this development. Figure 1 below shows where Heron fits into the overall Roadmap for QC in the IBM product system.
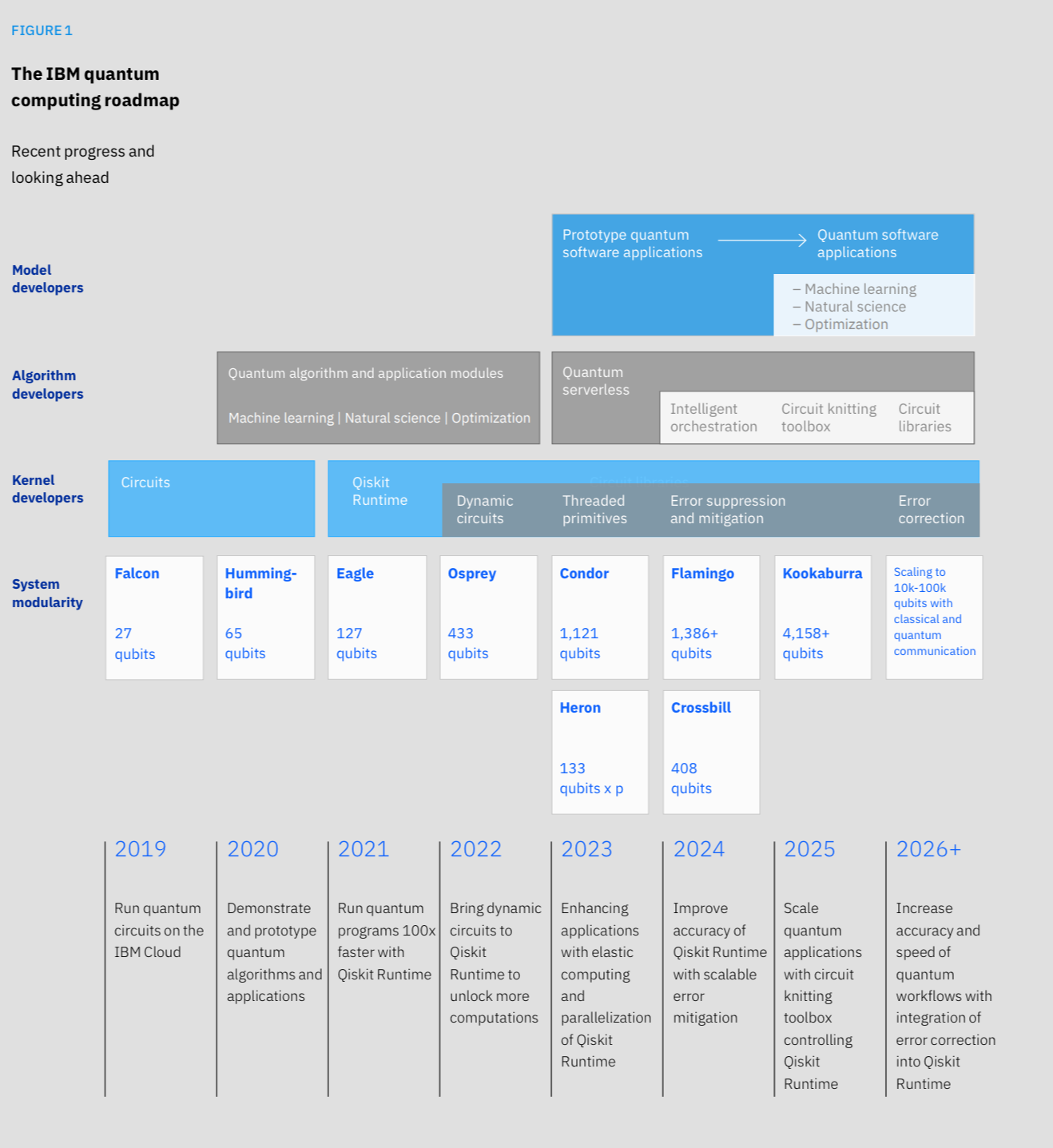
It is hoped that chips connected with conventional electronics, like fiber-optic or microwave connections, will pave the way for distributed, large-scale QC that can have as many as a million qubits connected to them simultaneously. It's possible that this is enough to run error-corrected quantum algorithms that are useful. The modularity of the system is essential, and the only way to scale quantum computing is to build modules with a few thousand qubits each and then begin linking those modules to one another in order to achieve coherent linkage.
Quantum Compute and AI Models
Quantum computing is a primary application of artificial intelligence (AI) applications, such as quantum-assisted models for generating data to enhance forecasting. Problems related to machine learning are pertinent to a variety of industries, including logistics, supply chian, automated trading, autonomous driving, speech and image recognition, and predictive maintenance, to name a few. Quantum computing has the potential to be an integral part of a larger movement toward democratizing artificial intelligence (AI) and opening the door for smaller businesses to train complex models theoretically at a fraction of the time and cost and without access to modern hyperscale data centers. This potential can be realized through the acceleration of machine learning training routines. The transition to AI applications that are able to process real-time data flows and that are continually improving or adjusting to circumstances presents an opportunity for businesses.
Quantum Computing and Logistics
The Quadratic Assignment Problem (QAP) is a complex combinatorial optimization problem used to optimize large combination problems which are especially common in logistics. Such as cargo and passenger traffic among aircraft, gates, personnel, and air traffic flows originating from and terminating at an airport. It is a challenging NP-hard class combinational optimization problem. The QAP was discovered in 1957 by Koopmans and Beckmann. It is an NP-Complete problem that can be applied to many other optimization problems outside of economics. The Koopmans-Beckman formulation of the QAP aims to achieve the objective of allocating facilities to locations so as to reduce the total cost. Koopmans and Beckmann introduced the computationally complex Koopmans-Beckman formulation of the QAP to optimize the transportation costs of goods between manufacturing plants and locations. Factoring in the location of each of the manufacturing plants as well as the volume of goods between locations to maximize revenue is what distinguishes this from other linear programming assignment problems like the Knapsack Problem.
Optimization of the QAP is easily stated but requires significant computational power. The number of potential facility/location combinations grows as n!
. Thus for a QAP of just n = 6
facilities and location, there are 720
facility/location pairings. The QAP can be used in applications beyond just the location of economic activities that it was originally developed for by Koopmans and Beckmann.
Experimental Findings
Experiments considering supply chain logistics and QC have demonstrated the practicality of variational algorithms for solving small-sized inventory control problems. The optimization of these routes is a complex process that must take into account a wide variety of outside factors. QC can provide larger inventory problem sets with real-time conditions of transportation and demand than classic compute. Through the optimization of shipping and delivery routes, it is possible to both anticipate and manage disruptions that occur during delivery. This is necessary due to the fact that supply chains frequently involve thousands of different business partners in addition to the presence of global risks and the requirement to maximize efficiency while simultaneously reducing costs. Currently a handful of companies are developing technology that might make it possible to boost network capacity and utilization while simultaneously lowering costs for trains, airlines and shipping.
The imperfect QC that are available today can be used to run a subset of applications that do not require an exact answer but instead place a greater emphasis on identifying patterns or a likely course of action. This, along with the fact that many companies that provide QC services now also provide cloud access, has made it possible for many more businesses to begin experimenting with quantum applications. QC is a primary application of artificial intelligence (AI) applications, such as quantum-assisted models for generating data to enhance forecasting.
Extrapolating to the Future
QC has the potential to be an integral part of a larger movement toward democratizing artificial intelligence (AI) and opening the door for smaller businesses to train complex models theoretically at a fraction of the time and cost and without access to modern hyperscale data centers. This potential can be realized through the acceleration of machine learning training routines. The transition to AI applications that are able to process real-time data flows and that are continually improving or adjusting to circumstances presents an opportunity for businesses.
Deploying Quantum Computing for Logistics
The current achievements in QC and their continued development are dependent on a number of enablers, including workforce readiness, standardization, and policy. Despite the amount of attention that is paid to these activities, these enablers are not yet in place.
Benchmarking Quantum Systems
As pointed out in the experiments conducted to test QC in logistics the hardware does not provide large enough compute to solve the gnarly problems industry is grappling with just yet. Because there is so much to gain from QC and even a small system provides substantial gains it is valuable for organizations to monitor QC to determine to what degree they can utilize it.
DARPA, having the same problem, developed metrics that can quantify the degree to which large QC will be useful or transformative once they become a reality. Re-inventing key quantum computing metrics, making those metrics testable, and estimating the required quantum and classical resources needed to reach critical performance thresholds are the goals of the DARPA Quantum Benchmarking project. In order to calculate the gap between the current state of the art and what is possible with quantum computing. This is a great project to utilize for substantial efforts in QC.
Along these lines the World Economic Forum has broken down the benchmarks to four general milestones that can be used to monitor QC on a more general application level. The basic milestones in the development of a quantum computing system are the same:
- The system must be able to create well characterized qubits.
- The system must allow for the qubits to be initialized, universally controlled and measurable for calculations.
- The system must be able to correct errors inherent in the physical hardware realization of the qubits.
- The system must be able to do all of the above at scale.
No systems today can operate at a scale (level 4) as of mid-2022. Currently, the most advanced platforms can handle a few hundred qubits. To achieve a true large scale, they would need to be able to control one million qubits - enough to show a real-life advantage in a quantum computation.
Real World Usage Considerations
When considering the QC system there are three main problems that need to be solved to build a quantum computer that can be used in the real world: scale, quality, and speed. These problems correspond to the areas where systems could be judged. So, the performance metrics can be roughly put into two groups: an analysis of each individual part and benchmarks that look at the whole system as a whole.
Architecture Design
Despite the fact that software and algorithm start-ups are expanding at a faster rate than hardware start-ups, more than 90 percent of investment went to hardware players in the year 2020. This means that QC is not user friendly and integration into existing systems will be challenging. Classical computers are anticipated to coexist with quantum ones for the foreseeable future. They will continue to power day-to-day activities for which QC are not optimally suited, and they will provide support for the processing workflow of QC.
QC are naturally positioned to work with complex systems where data has inherent structure and many variables. QC will make it possible for businesses to potentially improve their optimization and machine learning processes, which will allow them to discover new insights and make decisions that are both better and more precise. This is particularly important given the growing reliance that many industry sectors have on data for planning and operations. They are able to improve pattern recognition in data sets that are either structured or unstructured.
Maturity Model
Considering all the factors that go into selecting hardware and supporting it with software who uses QC and how far along are they? Overall not many are using it, but it is growing and some are further along than one would think.
McKinsey created an interesting and useful quantum computing maturity model that tracks maturity across five categories.
- Bystanders - they are not exploring QC (nothing has been released by them)
- Beginners - formed partnerships to explore QC use cases.
- Learners - several pre-competitive activities have moved to a competitive state and they hire QC scientists.
- Professionals - QC is impacting business and strategy is forming around it.
- Legends - Industry's innovation related to applications of QC has plateaued, but use cases continue to create value.
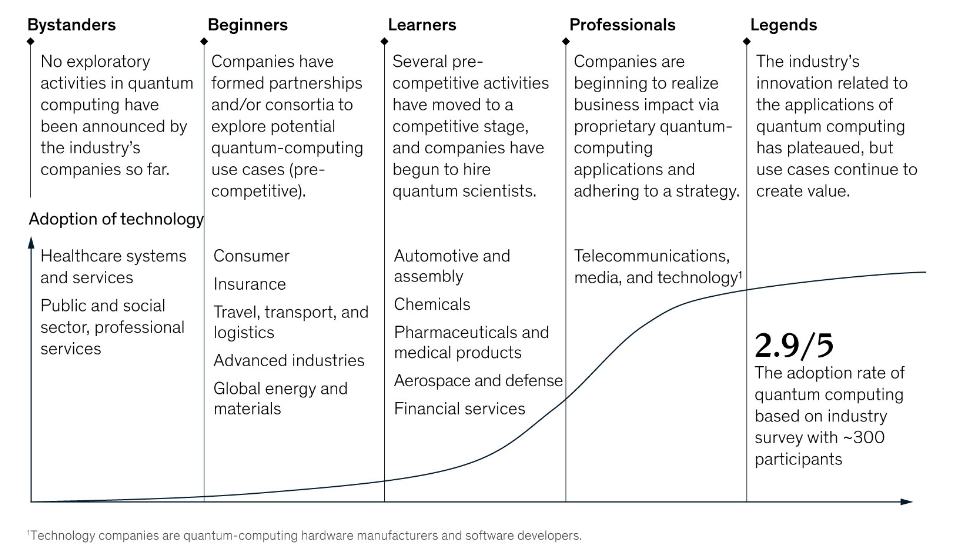
Companies in the fields of technology, media, and telecommunications have achieved a number of significant milestones over the course of the past five years, including:
- quantum supremacy
- development of an industrial quantum computer
- establishment of cloud-based quantum-computing services
These advancements mark important milestones on the road toward higher levels of quantum computing maturity.
It should be noted that the report give a grade of 2.9. This seems very high considering it is extremely hard to find use cases that are not laboratory demonstrations or technology companies preparing to build a QC to sell or lease. It is more common to hear of Learners building Quantum Algorithms and frameworks to potentially solve existing problems, but these seem to be in high value industries with established mathematical limitations to their problems.
References
- J. F. A. Sales and R. A. P. Araos, “Adiabatic Quantum Computing for Logistic Transport Optimization.” arXiv, Jan. 18, 2023. Accessed: May 25, 2023. [Online]. Available: http://arxiv.org/abs/2301.07691
- T. C. Koopmans and M. Beckmann, “Assignment Problems and the Location of Economic Activities,” Econometrica, vol. 25, no. 1, p. 53, Jan. 1957, doi: 10.2307/1907742.
- Dr. Imed Othmani, Dr. Mariana LaDue, and Dr. Martin Mevissen, “Exploring quantum computing use cases for logistics,” IBM, Aug. 2022. Accessed: May 25, 2023. [Online]. Available: https://www.ibm.com/downloads/cas/GWDGROG7
- H. Mohammadbagherpoor et al., “Exploring Airline Gate-Scheduling Optimization Using Quantum Computers.” arXiv, Nov. 17, 2021. Accessed: May 25, 2023. [Online]. Available: http://arxiv.org/abs/2111.09472
- H. Jiang, Z.-J. M. Shen, and J. Liu, “Quantum Computing Methods for Supply Chain Management,” in 2022 IEEE/ACM 7th Symposium on Edge Computing (SEC), Dec. 2022, pp. 400–405. doi: 10.1109/SEC54971.2022.00059.
- M. Masum et al., “Quantum Machine Learning for Software Supply Chain Attacks: How Far Can We Go?” arXiv, Apr. 04, 2022. Accessed: May 25, 2023. [Online]. Available: http://arxiv.org/abs/2204.02784
- “State of Quantum Computing: Buiding a Quantum Economy,” World Economic Forum, World Economic Forum, Sep. 2022.
- Yulin Wu, Wan-Su Bao, et al. “Strong quantum computational advantage using a superconducting quantum processor,” Pysical Review Letters, vol. 127, no. 18.
Note
- McKinsey adoption curve is (c) 2023 McKinsey & Company
- IBM Quantum Roadmap is (c) 2022 IBM